This post is a technical note on methodological issues in using Census public use microdata for housing needs assessment. In a series of posts over the paste few weeks, I have reviewed available data on the size of the low income populations eligible for housing assistance. One post reviewed data on LMI (low and moderate income) population assembled for the CDBG program. A second reviewed studies that use Census data to compare need for low income housing to supply of low income housing. A third reviewed a new data source on affordable housing in Massachusetts, the Housing Navigator, and concluded that it offers a good metric of the supply of affordable housing that is consistent with available administrative data and with the Census. But I still want to explore further what the Census data have to say about affordable housing need. Future posts will do that. This post merely documents some methodological challenges and solutions in using Census public use microdata to identify low and moderate income populations using HUD Section 8 income criteria.
The following basic HUD concepts have been discussed in a previous post:
- “Area Median Income” (AMI)
- “Extremely Low Income” (ELI) below 30% AMI (with adjustments)
- “Very Low Income” (VLI) below 50% of AMI
- “Low Income” (LI) below 80% of AMI.
These concepts are defined by HUD according to administrative and statutory considerations. There are two mechanical challenges in using Census data to evaluate how these HUD terms apply these income limits to populations.
- HUD measures income levels in geographic areas called HUD Metropolitan Fair Market Rent/Income Limits Areas (HMFA). HMFAs are subparts of Metropolitan Statistical areas and in Massachusetts they can be defined as a list of cities and towns. Although Census data is published within multiple geographic boundaries (nation, state, county, Metropolitan Statistical Area), the Census does not publish data for HMFAs.
- HUD income levels do not correspond directly to any Census recognized income levels, although they are derived from basic Census income data. There two kinds of difference:
- Temporal differences: HUD defines Area Median Income prospectively — for example, AMI in each HMFA for 2024 was effective April 1, 2024. Census data on income is released periodically and is retrospective. So HUD has to start from Census data from previous years and roll it forward based on inflation estimates. HUD also applies a series of accuracy tests and expands geographic scope to include enough population to achieve adequate accuracy. In a given HMFA, the AMI for 2024 is not necessarily going to be the same as the AMI based on census data collected in 2024.
- Conceptual differences: HUD applies a series of adjustments to compute income levels based on AMI — the area median income is just a starting point. These include comparisons to local rent levels, comparisons to poverty level, comparisons to statewide AMI, and restrictions on year to year change. In some cases, these adjustments are inapplicable. For example, in the Boston area in 2022, the HUD ELI/VLI/LI levels work out to be straight percentages of AMI. By contrast, in the Springfield area in 2022, multiple adjustments apply.
Deriving HMFA statistics from Census data
To apply geography concepts or income standards not present in standard census tabulations, one has to work with the American Community Survey Public Use Microdata — a downloadable set of records of individual survey interviews which can be recombined to produce tabulations not offered by the ACS table generator.
Microdata records are anonymized to protect privacy. To further protect privacy, no location identifiers are released for areas under 100,000 people. In the Public Use Microdata sample, records are geographically identified only by their state and “Public Use Microdata Area” or “PUMA.” Larger cities may include multiple PUMAs and smaller towns may be grouped together in a single PUMA, but, at least within Massachusetts, PUMAs do not split any communities — no PUMA contains a subpart of one community and a subpart of another community. For cross-referencing geographies, the Census endorses a tool called GeoCorr developed by the Missouri Census Data Center. Using this tool, one can extract a breakdown of each PUMA by city/town and the share of population or housing units contributed to the PUMA from each community. Since HUD also publishes a list of the communities in each HFMA, one can link these two files together to assign PUMAs through their comprising communities to HFMAs. The chart below lists the 54 PUMAs in Massachusetts, the HFMA which has the largest share of their housing units and the Metropolitan Statistical Area that the HFMA is part of. The Census also publishes reference maps for each PUMA in Massachusetts and a mapping tool to view Census boundary definitions.
Public Use Microdata Areas in Massachusetts (From 2020 Census), listed by Metropolitan Statistical Area and HUD HMFA
Metropolitan Statistical Area | HUD HMFA | Public Use Microdata Area | PUMA Code |
---|---|---|---|
Barnstable Town, MA | Barnstable Town, MA MSA | Barnstable (East), Dukes & Nantucket Counties–Outer Cape Cod Towns* | 1301 |
Barnstable Town, MA | Barnstable Town, MA MSA | Barnstable County (West)–Inner Cape Cod Towns & Barnstable Town City | 1201 |
Boston-Cambridge-Newton, MA-NH | Boston-Cambridge-Quincy, MA-NH | Boston City–Allston, Brighton & Fenway | 801 |
Boston-Cambridge-Newton, MA-NH | Boston-Cambridge-Quincy, MA-NH | Boston City–Back Bay, Beacon Hill, Charlestown, East Boston, Central & South End | 802 |
Boston-Cambridge-Newton, MA-NH | Boston-Cambridge-Quincy, MA-NH | Boston City–Dorchester & South Boston | 803 |
Boston-Cambridge-Newton, MA-NH | Boston-Cambridge-Quincy, MA-NH | Boston City–Hyde Park, Jamaica Plain, Roslindale & West Roxbury | 805 |
Boston-Cambridge-Newton, MA-NH | Boston-Cambridge-Quincy, MA-NH | Boston City–Mattapan & Roxbury | 804 |
Boston-Cambridge-Newton, MA-NH | Boston-Cambridge-Quincy, MA-NH | Essex County (Central)–Peabody, Danvers & Saugus | 704 |
Boston-Cambridge-Newton, MA-NH | Boston-Cambridge-Quincy, MA-NH | Essex County (North)–Newburyport, Amesbury & North Andover* | 703 |
Boston-Cambridge-Newton, MA-NH | Boston-Cambridge-Quincy, MA-NH | Essex County (South Coastline)–Salem, Beverly & Gloucester | 706 |
Boston-Cambridge-Newton, MA-NH | Boston-Cambridge-Quincy, MA-NH | Essex County–Lynn & Nahant | 705 |
Boston-Cambridge-Newton, MA-NH | Boston-Cambridge-Quincy, MA-NH | Middlesex County (East)–Cambridge | 611 |
Boston-Cambridge-Newton, MA-NH | Boston-Cambridge-Quincy, MA-NH | Middlesex County (East)–Malden & Medford | 609 |
Boston-Cambridge-Newton, MA-NH | Boston-Cambridge-Quincy, MA-NH | Middlesex County (East)–Somerville & Everett | 610 |
Boston-Cambridge-Newton, MA-NH | Boston-Cambridge-Quincy, MA-NH | Middlesex County (Southwest) | 606 |
Boston-Cambridge-Newton, MA-NH | Boston-Cambridge-Quincy, MA-NH | Middlesex County (West Central) | 604 |
Boston-Cambridge-Newton, MA-NH | Boston-Cambridge-Quincy, MA-NH | Middlesex County–Framingham & Marlborough | 605 |
Boston-Cambridge-Newton, MA-NH | Boston-Cambridge-Quincy, MA-NH | Middlesex County–Lexington, Burlington & Wilmington | 607 |
Boston-Cambridge-Newton, MA-NH | Boston-Cambridge-Quincy, MA-NH | Middlesex County–Newton & Waltham | 613 |
Boston-Cambridge-Newton, MA-NH | Boston-Cambridge-Quincy, MA-NH | Middlesex County–Watertown, Arlington & Belmont | 612 |
Boston-Cambridge-Newton, MA-NH | Boston-Cambridge-Quincy, MA-NH | Middlesex County–Woburn, Melrose & Reading | 608 |
Boston-Cambridge-Newton, MA-NH | Boston-Cambridge-Quincy, MA-NH | Norfolk County (Central)–Norwood, Stoughton & Canton | 905 |
Boston-Cambridge-Newton, MA-NH | Boston-Cambridge-Quincy, MA-NH | Norfolk County (Northeast)–Weymouth, Braintree, Randolph & Cohasset | 904 |
Boston-Cambridge-Newton, MA-NH | Boston-Cambridge-Quincy, MA-NH | Norfolk County (Northwest)–Needham, Wellesley & Westwood | 901 |
Boston-Cambridge-Newton, MA-NH | Boston-Cambridge-Quincy, MA-NH | Norfolk County (Southwest)–Franklin, Walpole & Foxborough | 906 |
Boston-Cambridge-Newton, MA-NH | Boston-Cambridge-Quincy, MA-NH | Norfolk County–Brookline, Milton & Dedham | 902 |
Boston-Cambridge-Newton, MA-NH | Boston-Cambridge-Quincy, MA-NH | Norfolk County–Quincy | 903 |
Boston-Cambridge-Newton, MA-NH | Boston-Cambridge-Quincy, MA-NH | Plymouth County (North)–Marshfield, Hingham & Scituate | 1103 |
Boston-Cambridge-Newton, MA-NH | Boston-Cambridge-Quincy, MA-NH | Plymouth County (South)–Plymouth Town, Middleborough & Wareham* | 1104 |
Boston-Cambridge-Newton, MA-NH | Boston-Cambridge-Quincy, MA-NH | Suffolk County (North)–Revere, Chelsea & Winthrop | 806 |
Boston-Cambridge-Newton, MA-NH | Brockton, MA | Plymouth County (West)–Bridgewater, Abington & Whitman | 1102 |
Boston-Cambridge-Newton, MA-NH | Brockton, MA | Plymouth County–Brockton | 1101 |
Boston-Cambridge-Newton, MA-NH | Lawrence, MA-NH | Essex County (Northwest)–Haverhill & Methuen | 701 |
Boston-Cambridge-Newton, MA-NH | Lawrence, MA-NH | Essex County (West)–Lawrence & Andover | 702 |
Boston-Cambridge-Newton, MA-NH | Lowell, MA | Middlesex County (Northeast)–Billerica, Tewksbury & Dracut | 603 |
Boston-Cambridge-Newton, MA-NH | Lowell, MA | Middlesex County (Northwest)–Outside Lowell | 601 |
Boston-Cambridge-Newton, MA-NH | Lowell, MA | Middlesex County–Lowell | 602 |
Pittsfield, MA | Pittsfield, MA | Berkshire County–Pittsfield* | 100 |
Providence-Warwick, RI-MA | New Bedford, MA | Bristol County (South)–New Bedford, Dartmouth & Westport | 1004 |
Providence-Warwick, RI-MA | Providence-Fall River, RI-MA | Bristol County (Central)–Fall River, Somerset & Acushnet | 1003 |
Providence-Warwick, RI-MA | Providence-Fall River, RI-MA | Bristol County–Attleboro, North Attleborough & Swansea | 1001 |
Providence-Warwick, RI-MA | Taunton-Mansfield-Norton, MA | Bristol County–Taunton, Easton & Mansfield | 1002 |
Springfield, MA | Franklin County, MA | Franklin & Hampshire (West, North, East) Counties* | 201 |
Springfield, MA | Springfield, MA | Hampden County (Central)–Springfield | 402 |
Springfield, MA | Springfield, MA | Hampden County (East)–Chicopee | 403 |
Springfield, MA | Springfield, MA | Hampden County (West)–Westfield & Holyoke | 401 |
Springfield, MA | Springfield, MA | Hampshire County (South)–Northampton | 301 |
Worcester, MA-CT | Fitchburg-Leominster, MA | Worcester County (Northeast)–Fitchburg, Leominster & Lunenburg | 502 |
Worcester, MA-CT | Fitchburg-Leominster, MA | Worcester County (Northwest)–Gardner* | 501 |
Worcester, MA-CT | Worcester, MA | Worcester City (East) | 504 |
Worcester, MA-CT | Worcester, MA | Worcester City (West) | 505 |
Worcester, MA-CT | Worcester, MA | Worcester County (East Central)–Outside Worcester City* | 503 |
Worcester, MA-CT | Worcester, MA | Worcester County (South) | 507 |
Worcester, MA-CT | Worcester, MA | Worcester County (West Central)–Outside Worcester City | 506 |
When computing income limits using this data, on has to decide how precise to be geographically:
- Most precisely, one could compute the limits for each HFMA-overlap component of the PUMA and weight them to derive an income limit for the PUMA.
- One could just compute the limit for the majority HFMA and use that for the PUMA. Some PUMAs overlap more than one HFMA, but of 54 Massachusetts PUMAs, 38 are are in only one HFMA, another 9 are over 75% in one HFMA and the remaining 7 are over 48% in one HFMA. This approach has the value that computations can be checked directly for exact match to published limits.
- One could further approximate by computing an income limit for the whole MSA and using that for all the PUMAs that lie majority within the MSA. It turns out that approximating to the MSA level rolls every PUMA into a single area — the only exception being the Outer Cape Cod Towns PUMA, which includes the Vineyard and Nantucket as well as towns on the Cape. The Vineyard is its own Micropolitan Statistical Area and Nantucket is outside any Metro or Micro Statistical Area.
The choice about geographical precision goes along with the choice about income level precision.
Developing Income Levels for Application to Census Data
Our primary goal in using American Community Survey data is to estimate the size of the population at different HUD income eligibility levels. The official HUD computation of these income levels is complex and one has to decide how much of that complexity to match as one applies income levels to each household in the microdata. Options include:
- Writing code to look up the published HUD level for each household. The levels for each household size in each HFMA are available for download. However, the HUD levels are published only for household sizes of 8 or below. The ACS data for Massachusetts does include some larger households (up to 20 persons).
- A hybrid coding approach looks up the HUD-computed income levels for a 4-person household and applies rules to determine the household size adjustments. For the ELI level, one needs to also apply the rule that the ELI level is not less than the poverty level and not more than the VLI level. This approach turns out to compare perfectly to the HUD-computed levels (see attached verification spreadsheet), because all of the complex HUD income level rules are applied to set the 4-person level and then the only remaining computation is family-size adjustment. [One exception to this straightforwardness is that for the ELI level, cap and floor rules checked again because of the poverty rule, but this does not appear to have affected 2022 level computations in MA.] As noted previously, even with complete precision on the income level, one may choose to approximate geographically by using just the majority HFMA or MSA for the PUMA instead of weighing all HFMA components. Using the majority HFMA approach has the advantage that all PUMAs will have levels that match to published HFMA levels — weighting introduces a lot of minor variation.
- A third approach is to approximate the HUD computation by setting the levels as if they were simply percentages (30/50/80) of the Census-published median family income for the containing MSA (and then applying household size adjustment). This triple approximation — as to timing (retrospective Census or prospective HUD), formula, and geography — is reasonable and saves a lot of steps because the ACS does publish retrospective median incomes at the MSA level. This appears to be the approach used in the GAP study by the National Low Income Housing Coalition. It yields ELI/VLI population counts at the statewide level that are not too different form those derived more precisely from HUD rules. One could optionally apply the HUD rule requiring the ELI to be above the poverty level, but not greater than the VLI level.
- A fourth approach, applicable only to the ELI computation, is to approximate the ELI level on a statewide basis as a ratio to the poverty level. Using a ratio to poverty level has the advantage that one can readily compare results to published ACS tables which does include comparisons of household income to poverty level. This approach is the roughest since it does not vary at all by geography within the state (poverty level is set nationally without regard to state or local variation in cost of living). One could estimate a ratio just by comparing poverty levels to HUD income limits (see some experimentation with this approach in an earlier post), but one could set a ratio with confidence only by comparing the results of the poverty ratio approach to the results of one of the other methods. And one would have to be concerned that the ratio would diverge from reality over time.
The choice between the first two approaches is purely a logistical one. But the third and fourth approaches, while greater offering logistical simplification, also bypass HUD’s computations, which may make sense. HUD’s computations are constrained by various administrative considerations — like not changing levels too rapidly which could distress either owners or tenants. Following HUD’s approach makes sense for some purposes — like comparing available housing supply to the population eligible under current HUD rules. But for assessment of overall distribution of need, it may make sense to look also at the MSA approximation approach, the third approach.
Results of Alternative Approaches
It turns out that the statewide count of households at the three income income levels — ELI, VLI, LI — is not terribly sensitive to the variations in definition approach discussed above, at least in the dataset we are using: the American Community Survey 2022 5-year Public Use Microsample. The charts below explore several combinations of the geographic and income-level options discussed above. The first shows how the ELI level varies under several different definitions for several particular PUMAs.
4-person household Extremely Low Income Level in 2022 ACS 5-year sample under alternative methods in three Public Use Microdata Areas
Geography and income level computation method | Springfield (401) | Allston, Brighton & Fenway (802) | Barnstable (East) with Islands (1301) |
---|---|---|---|
(1) HUD Income Levels, weighted with PUMA overlap to HFMA | $28,250 | $42,050 | $34,182 |
(2) HUD Income Levels, using HFMA containing majority of PUMA | $28,250 | $42,050 | $32,600 |
(3) Income levels based on percentages of Family Median for MSA | $27,700 | $40,400 | $35,600 |
(4) Income levels based on percentages of Family Median for MSA with rule that ELI cannot be less than poverty level | $27,750 | $40,400 | $35,600 |
(5) Income levels based on percentages of Family Median for MSA with rule that ELI cannot be less than poverty level with adjustment for MA living costs | $30,359 | $40,400 | $35,600 |
Some observations about the ELI levels in the table above:
- The first two lines match the official HUD ELI levels for 2022, except that Method 1 is slightly higher for Barnstable because it weighs in the higher ELIs for Nantucket and the Vineyard which are separate (high value) HUD market areas, yet combined within the Barnstable PUMA.
- Methods 3, 4, and 5 yield the same ELI for Allston/Brighton/Fenway and for Barnstable because their family area median income is so high that 30% of it is greater than the poverty level, so factoring in the poverty level as a minimum (even with the 9.4% upward adjustment in Method 5) makes no difference.
- Springfield shows an increase in ELI from Method 3 to Method 4 to Method 5 because its Area Family Median Income is lower so the poverty level minimum applies.
The next chart shows the limited variation in the statewide household counts resulting from the several methods.
Statewide Massachusetts renter households at income levels in 2022 ACS 5-year sample under alternative methods
Geography and income level computation method | ELI | VLI not ELI | LI not VLI | Not LI |
---|---|---|---|---|
(1) HUD Income Levels, weighted with PUMA overlap to HFMA | 309,765 | 164,147 | 191,186 | 368,313 |
(2) HUD Income Levels, using HFMA containing majority of PUMA | 308,082 | 163,518 | 191,561 | 370,250 |
(3) Income levels based on percentages of Family Median for MSA | 309,863 | 163,820 | 191,307 | 368,421 |
(4) Income levels based on percentages of Family Median for MSA with rule that ELI cannot be less than poverty level | 310,388 | 163,295 | 191,307 | 368,421 |
(5) Income levels based on percentages of Family Median for MSA with rule that ELI cannot be less than poverty level with adjustment for MA living costs | 311,289 | 162,394 | 191,307 | 368,421 |
Notes to Lines in the Previous Two Tables
- Applying official HUD income levels for each HUD-defined HFMA. Where a PUMA lies across multiple HFMA, apply the weighted average of the overlapping HFMA levels, with weighting by household count.
- Applying official HUD income levels for each HUD-defined HFMA. Where a PUMA lies across multiple HFMA, use the majority HFMA value. In the 2012 PUMA definitions (applicable to survey years 2018 through 2021), PUMA 400, “Worcester & Middlesex Counties (Outside Leominster, Fitchburg & Gardner Cities)”, is split 7 ways across HFMA’s; in this instance we used the HFMA with the second largest overlap, which is with the Fitchburg-Leominster, MA HUD Metro FMR Area, because it makes more sense geographically — this PUMA wraps around Fitchburg and Leominster.. (Additional 2010 PUMA maps here.)
- In this computation, we mapped PUMAs to the Core Based Statistical Area (Metropolitan or Micropolitan) that contained the majority of their households. Because the CBSAs are larger than HFMAs, most PUMAs lie entirely within one CBSA. We then derived income levels as straight percentages (30/50/80) of the ACS Reported Median Family Income in the last 12 month for the CBSA (applying household size adjustments as HUD does). As noted above, this appears to be the method used by the National Low Income Housing Coalition, although they get a slightly higher count for ELIL renter households, 316,201; see the methodology section of their Gap Report.
- This is a variation of the previous computation, adding in a rule that the ELIL cannot be less than the federal poverty guideline or more than the VLIL.
- This is a variation of the previous computation, but adjusting the federal poverty guideline upward by a regional purchasing price adjustment from the Bureau of Economic Analysis (1.094)
In all methods, household size adjustment followed HUD practice using this algorithm.
Public Function Base_Person(ByVal Base As Double, ByVal Person As Double) As Double
' Person is the Household Person count
' Base is the applicable median family income for a 4 person household
Base_Person = Ceiling(Base * IIf(Person < 5, 1 - (4 - Person) * 0.1, 1 + (Person - 4) * 0.08), 50)
End Function
Poverty level was computed by household size using this algorithm (with an inflation adjustment in Method 5).
Public Function Poverty_22(ByVal Person As Double) As Double
' Person is the Household Person count
Poverty_22 = 13590 + (Person - 1) * 4720
End Function
All counts include two tenure types: “Rented” and “Occupied without payment of rent.” Owner occupant households are excluded from the count. These choices will be explored in a future post.
See attached spreadsheets for computation details.
There is only a 1% difference in the statewide Extremely Low Income household count across all the methods above. It makes sense that the ELI count is not too sensitive to the ELI threshold, because ELI households mostly have incomes well below the ELI threshold (which was $24,950 statewide in 2022 for a 1-person households) as shown in the graphic below.
Single Person Renter Households in ACS 2022 5-year sample by income in past 12 months
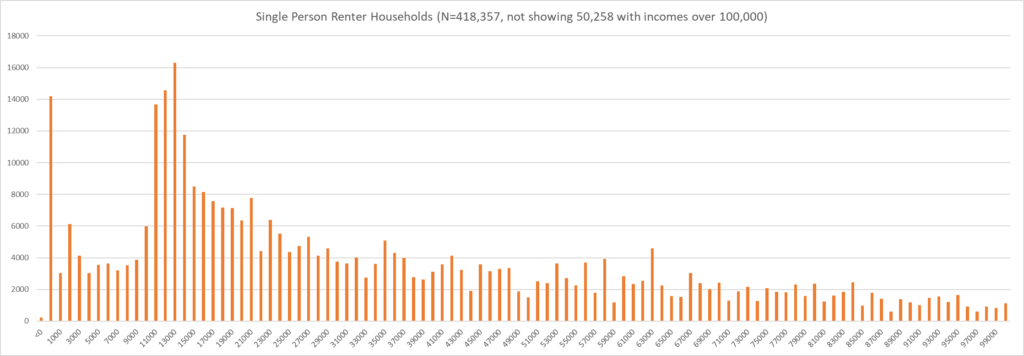
The differences across the methods are, however, somewhat larger when comparing within individual HMFAs. The differences can run in different directions as a result of conflicting dynamics:
- Moving from an HFMA as a geographic unit to the larger MSA that contains it can raise or lower the applicable AMI. The Brockton, Lawrence, and Lowell HFMA’s all have AMI’s lower than the AMI for the larger “Boston-Cambridge-Newton, MA-NH Metro Area” which contains them. But the Boston HFMA has a slightly higher AMI than that larger containing MSA.
- In Method 3, there is no rule applying the poverty guidelines as a minimum for the ELI, so the ELI level can work out to be lower than in Methods 1 and 2. Conversely, in Method 5, an upwards-adjusted poverty level is applied. But the poverty adjustment makes a difference only for larger families. For single person families, the regional household-size-adjusted AMI is always higher. Household size adjustments are included in both HUD’s income levels and the federal poverty guidelines, but HUD’s adjustment method is more generous to small households and less generous to large households than the poverty guidelines. The HUD adjustment method derives the 1-person level as 70% of the 4-person level, while in 2022 under the federal poverty guideline the 1-person level works out (through a completely different computation) to be 49% of the 4-person level.
These dynamics are reflected in the chart below. The chart compares results from Methods 2 and 5 (the lowest and highest statewide totals) and shows that:
- The lower income HFMAs within the larger “Boston-Cambridge-Newton, MA-NH Metro Area” — Brockton and Lawrence — show an increase in Method 5. For smaller households, this is driven by using the higher metropolitan AMI. Larger households, get a higher ELI in Method 5 because of the poverty threshold adjustment.
- In the statewide total, a decrease in ELI households in the Boston HFMA offsets the Brockton/Lawrence increase: In the Boston HFMA, the “Boston-Cambridge-Quincy, MA-NH HUD Metro FMR Area,” the AMI is high — $140,200. As a result, the rule that the ELIL threshold must be above the poverty line is not engaged. However, the larger “Boston-Cambridge-Newton, MA-NH Metro Area” AMI is a little lower at $134,646, so Method 5’s computation shows fewer households at the ELI level.
- Springfield combines the above dynamics in an especially confusing way: The Springfield HFMA’s ELI count goes down slightly from Method 2 to Method 5 in the table above, while the first table in this section shows the ELI level definition going up. This is because the first table is showing the ELI for a 4 person family, while the table below is showing the count for all family sizes — the ELI level definition for single person families is actually highest in Method 2. Method 2 and Method 5 both use the HUD household size adjustment which results in an AMI-based computation of ELI that is well above the poverty threshold for the 1-person household in Springfield. However, Method 2 reflects some HUD AMI adjustments which push VLI and ELI upward for Springfield, so it results in a higher 1-person ELI, contributing to the slight decrease from Method 2 to Method 5 shown in the table above. For the 4-person ELI in the first table, the adjusted poverty minimum in Method 5 controls the ELI.
Count of Extremely Low Income Renter households by HFMA in Massachusetts — Method 2 compared to Method 5
HFMA | Method 2 ELI Count | Method 5 ELI Count | Pct Diff |
---|---|---|---|
Barnstable Town, MA MSA | 5,639 | 6,208 | 10% |
Boston-Cambridge-Quincy, MA-NH HUD Metro FMR Area | 170,197 | 165,122 | -3% |
Brockton, MA HUD Metro FMR Area | 9,276 | 11,631 | 25%* |
Fitchburg-Leominster, MA HUD Metro FMR Area | 7,659 | 8,763 | 14% |
Franklin County, MA HUD Metro FMR Area | 4,506 | 4,395 | -2% |
Lawrence, MA-NH HUD Metro FMR Area | 14,265 | 16,690 | 17%* |
Lowell, MA HUD Metro FMR Area | 11,845 | 12,501 | 6% |
New Bedford, MA HUD Metro FMR Area | 9,570 | 10,762 | 12% |
Pittsfield, MA HUD Metro FMR Area | 5,037 | 4,671 | -7% |
Providence-Fall River, RI-MA HUD Metro FMR Area | 11,339 | 12,365 | 9% |
Springfield, MA HUD Metro FMR Area | 28,371 | 28,327 | 0% |
Taunton-Mansfield-Norton, MA HUD Metro FMR Area | 4,829 | 4,618 | -4% |
Worcester, MA HUD Metro FMR Area | 25,549 | 25,236 | -1% |
TOTAL, Statewide | 308,082 | 311,289 | +1% |
Statistical confidence testing
The microdata used to produce the estimates above reflect only a sample of the population, so sampling error could skew any of the estimates above. .
Sampling error is the uncertainty associated with an estimate that is based on data gathered from a sample of the population rather than the full population. Note that sample-based estimates will vary depending on the particular sample selected from the population. Measures of the magnitude of sampling error, such as the variance and the standard error (the square root of the variance), reflect the variation in the estimates over all possible samples that could have been selected from the population using the same sampling methodology.
Chapter 12: Variance Estimation in the ACS methodology documentation.
In the ACS public use micro sample, each household interview record includes a weight. The population estimate for households meeting some criteria (for example, being ELI under one of the definitions above) is derived by summing the weights for the records meeting the criteria. (The weighting process is explained in Chapter 11 of the Metholodogy report.) In addition to the base estimate weights, the ACS microdata include for each record a set of 80 replicate weights that effectively simulate resampling of the population, so allowing an estimate of the standard error. As indicated in the formula below, one recomputes a population estimate using each of the replicate weights and derives the variance as the sum of the squares of the differences between the 80 estimates based on replicate weights and the original estimate. In the formula below, ?0 is the original estimate based on the household weights and the variable ?r runs across the estimates based on the replicate household weights.
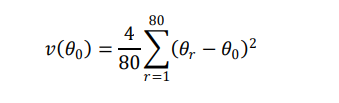
The standard error of the estimate, SE(?), is the square root of the variance, v(?0), shown above. A 90% confidence interval for an estimate is the estimate plus or minus 1.645 * the SE. For our statewide estimates of extremely low income renter households using alternative methodologies, the SE’s all work out to approximately 3,000, so the 90% confidence interval is +/- approximately 5,000. So, the variations of results from our refinements of income methodology are much smaller than the expected sampling error at the state-wide level.
Reviewing the HFMA level differences and using the Z test of statistical significance of the difference, most of the differences are not significant: Only the Brockton and Lawrence differences are statistically significant at the p<.05 level; the New Bedford difference is significant at the 0.1 level.
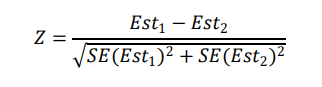
Discussion
One can make arguments for using Method 5 above: Income levels based on percentages of Family Median for MSA with the rule that the ELI level cannot be less than poverty level with adjustment for MA living costs. This method has the following advantages:
- It is simple to generate but approximates HUD income levels on a statewide basis.
- By grouping income levels at the Metropolitan level it treats the state housing market in a less fragmented way — there is substantial mobility within the large Boston MSA. We hear anecdotal reports of families moving from Boston or Chelsea to Brockton or Lawrence to lower housing costs.
- It yields the highest ELI count (although not very different from the HUD-based ELI count). To the extent that in future analysis, we are going to look at potential exclusions from the ELI population (like students and people with assets), starting from a higher base count of ELI is conservative.
- The HUD income level rules are designed with administrative concerns in mind — like avoiding a sudden increase or decrease in rent. These concerns not central to assessment of overall housing need.
However, at the end of the day, the refinements of income level computation turn out not to be important. While we were able to exactly replicate HUD income level computations in Massachusetts for 2022 and to test alternatives to those computations, none of the refinements rise much above the statistical noise level. In fact, setting levels simply using the statewide median family income from the census (122,530) without any geographic variation or HUD market refinements yields an ELI count (316,074) which is not statistically different from Method 5, and differs from Method 2 only at the .1 significance level.
What does make a big difference is the choice to follow the HUD household size adjustment method which (as discussed above) is more generous to small households than the poverty household size adjustment method. Preserving the 4-person ELI level at 30% of median income, but using the poverty household size ratios cuts the ELI count to 257,990. While the merits of the alternative household-size ratios are debatable, the choice of the HUD ratios makes sense for consistency in the housing context.
The table “Massachusetts households at various income levels 2022 ACS 5-year sample under alternative methods” should read “Massachusetts **renter** households at various income levels 2022 ACS 5-year sample under alternative methods” because it does not include households in owner occupied units that are within the income groups.
Correct! Fixed. Thank you for the careful read. I have updated other aspects of the discussion following this table in the past 24 hours.